PROJECT OVERVIEW
Measuring the impact of AI tools in government
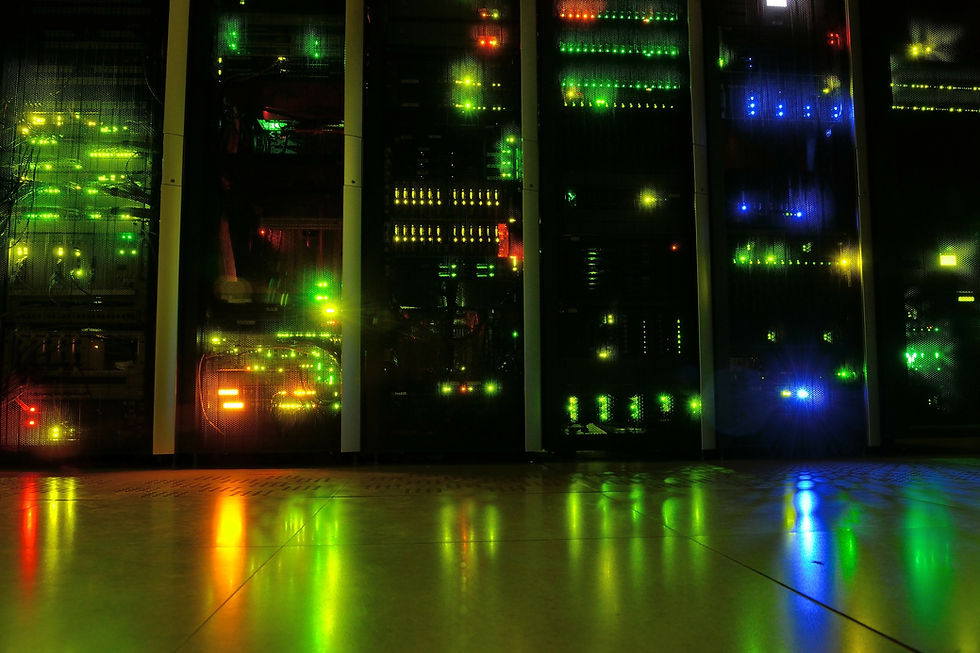
Highlights
Given the complexity of rules in safety net programs, there’s a lot of opportunity for Large Language Model-based tools to help. For example, for SNAP in 2022, 17% of terminations, suspensions, denials made in error and about 2 in 5 cases contained some kind of procedural error.
How can we ensure that how these tools are implemented will actually reduce administrative burdens and disparities in program access?
We’re developing a methodology for evaluating the use of these tools, guided by research on Administrative Burdens and Algorithmic Fairness. We’ll be working with Nava PBC to assess their use of ChatGPT 4 to assist hundreds of benefits navigators in CA.
Overview
The project goal is to evaluate, using a replicable approach, whether LLMs ought to be applied to specific use cases within public services, and provide structured summaries of findings (including performance, efficiencies, risks, and reductions in administrative burdens) for decision-makers to use when weighing LLM adoption.
The hope to produce a summary of trade-offs that can assist decision-makers at public agencies to assess whether adopting of Large Language Model (LLM)-based tools to assist staff aligns with their mission, considering factors such as:
Accuracy of actions staff take
Cost of development and fine-tuning
Training staff
Ensuring disparities are not exacerbated
Approach
Run an off-line randomized controlled trial with 3,600 scenarios to assess the adoption of LLMs using nationally representative circumstances, oversampling for demographic correlates of errors. Responses in the form of a next step or decision are collected from three experimental conditions: a hypothetical LLM-only condition, a human-only condition, and a human supported by LLM condition. The LLM tool itself is fine-tuned on similar question-and-answer data regarding government services, and was created by Nava using ChatGPT 4. We recruited retired SNAP quality control auditors to assist in designing questions and providing correct answers.

Image Caption: We use SNAP QC data to tnform evaluation areas
Funders: NSF (#2427748), Walmart Foundation, TPP
Timeline
October 2024 - Current
In Progress
Programs
SNAP
Topics
Large Language Models (LLMs), Artificial Intelligence, RCT, Algorithmic Fairness